Introduction
Approximately one third of our lives is spent sleeping. Good quality sleep is essential for good health and well-being.
The main effects of sleep deprivation include physical effects (sleepiness, fatigue, hypertension), cognitive impairment (deterioration of performance, attention and motivation; diminishment of mental concentration and intellectual capacity, and increase of the likelihood of accidents at work and during driving) and mental health complications. Inadequate rest impairs the ability to think, to handle stress, to maintain a healthy immune system and to moderate emotions (World Health Organization, 2004, p. 2).
According to Buysse, Reynolds, Monk, Berman, and Kupfer (1989) ‘sleep quality’ is an important clinical construct because complaints about sleep are common (15 – 35% of the adult population). Furthermore, sleep disturbances and complaints are associated with anxiety and stress (Karacan, Thornby, & Williams, 1983) and are frequently reported in psychiatric disorders like depression and schizophrenia (Buysse et al., 1989).
Different tools for measuring various aspects of sleep are described by Beck, Schwartz, Towsly, Dudley, and Barserick (2004). These include polysomnography, actigraphy and self-reports. Many self-report instruments are reported in the literature. These include the Pittsburgh Sleep Quality Index (Buysse et al., 1989), the Verran and Snyder-Halpern Sleep Scale (Snyder-Halpern & Verran, 1987) and the Karolinska Sleep Diary (Akerstedt, Hume, Minors, & Waterhouse, 1994).
The Pittsburgh Sleep Quality Index (PSQI)
The PSQI is one of the best known self-rating instruments used to measure sleep quality. The PSQI items were derived from three sources: clinical intuition and experience with sleep disorder patients, a review of other sleep quality questionnaires and clinical experience with the instrument during 18 months of field work (Buysse et al., 1989). It consists of 18 self-rating questions assessing a wide variety of factors relating to sleep quality grouped into 7 components (subjective sleep quality, sleep latency, sleep duration, habitual sleep efficiency, sleep disturbances, use of sleeping medications and daytime dysfunction). Each component is scored separately, weighted equally on a 0 – 3 scale. The scores of the 7 components are then added to give a global PSQI score, which has a range of 0 – 21 with higher scores indicating worse sleep quality. The 18 scale items are structured so that three of the components are measured by one item, two components by two, one component by three and one by nine items (one item is used for the calculation of two component scores).
The PSQI shares similarities with other instruments but Buysse et al. (1989) emphasize the following three important differences. First, the PSQI assesses the quality of sleep over the previous month. Second, other studies (such as Beutler, Thornby, & Karacan, 1978; Domino, Blair, & Bridges, 1984; Webb, Bonnet, & Blume, 1976) have used factor analysis to generate specific factors, whereas the PSQI components are empirical and clinical in origin rather than statistical. Third, there are differences in the scoring; the PSQI assigns ordinal scores to quantitative and qualitative information, allowing for the generation of component scores and a single global score giving a single overall assessment of sleep quality, being simple to calculate and allowing for direct comparisons of individual patients or groups. The last two differences, although intended to be advantages of this particular scale, are perhaps weakness in that the structure of the scale has never been fully investigated and it is not always easy to assign ordinal scores to qualitative data. Furthermore, the scoring of the scale is perhaps more complicated than intended. Finally, the PSQI was designed to assess clinical samples, while most previous questionnaires (such as in Bixler, Kales, Soldatos, Kales, & Healy, 1979; Karacan et al., 1983) have been designed to assess normal sleep habits of entire populations. The researchers feel that an investigation into the appropriateness of the scale on a non-clinical population is long overdue.
Studies on the Validity and Reliability of the PSQI
In the original study of the PSQI (Buysse et al., 1989), the reliability of the instrument was supported by Cronbach’s alpha for the whole 18-item questionnaire and for the 7 component scores. Also test-retest correlations of the global scores and the component scores were reported and statistical tests performed on the global scores and on the component scores from the two different administrations of the instrument leading to the conclusion of stable responses across time.
The degree of validity of the instrument was supported by detection of differences between distinct groups in the sample (healthy control subjects with no complaints about sleep, patients with major depressive disorder and patients with disorder of initiating and maintaining sleep). Also concurrent polysomnographic findings were obtained and finally correlations between global score and component scores were reported.
Since then, many studies have focused on the reliability and validity of the PSQI. Among them, there are studies with samples of elderly people (Buysse, Reynolds, Monk, Berman, & Kupfer, 1991), patients with AIDS (Rubinstein & Selwyn, 1998), patients with panic disorders (Stein, Chartier, & Walker, 1993), cancer patients (Beck et al., 2004), patients with primary insomnia (Backhaus, Junghanns, Broocks, Riemann, & Hohagen, 2002), bone marrow transplant patients and women with breast cancer (Carpenter & Andrykowski, 1998) and Nigerian students (Aloba, Adewuya, Ola, & Mapayi, 2007).
The most commonly reported index of reliability (internal consistency) is Cronbach’s alpha (Cronbach, 1951). The evidence collected to investigate the degree of validity of the PSQI commonly found in the literature includes correlations of component scores with global scores and comparisons of scores of distinct groups typically identified as having poor quality of sleep with control groups. Examples of such experimental groups include insomnia patients (Backhaus et al., 2002; Aloba et al., 2007), patients with psychiatric disorders (Doi et al., 2000), and low and high fatigue cancer patients (Beck et al., 2004). Finally, for the validation of the scale, correlations between PSQI global scores and related (or unrelated) constructs were calculated (Carpenter and Andrykowski, 1998) and comparisons were made between PSQI scores and daily logs (Backhaus et al., 2002).
No matter what instruments are used in measuring quality of sleep, good measurement is essential for further investigating the validity, reliability and appropriateness of the level of scaling of the instrument in relation to any given target population.
Rasch Measurement
Rasch models were originally designed for use in educational assessment. However, over recent years there has been an increase in their use in health and social research. The reason for this new interest is the ability of these models to handle reliability and validity issues, to refine questionnaires by improving category functioning and by decreasing the number of items while retaining the psychometric properties of the instruments. In order to show the diversity of situations where the models can be used productively, Panayides, Robinson, and Tymms (2010) reported a selection of applications of Rasch measurement. For example, Prieto, Roset, and Badia (2001), assessed the psychometric properties of the Spanish version of the assessment of growth hormone deficiency in adults. Massof and Fletcher (2001) assessed and refined the visual functioning questionnaire. Myford and Wolfe (2002) identified and resolved discrepancies in examiners’ ratings. Chen, Bezruczko, and Ryan-Henry (2006) described mothers’ effectiveness in caregiving for their adult children with intellectual disabilities. More recently, Panayides and Walker (2012) refined a widely used Internet Addiction scale using these models.
Of great importance in the validation process of any instrument is its factor structure. Panayides and Walker (2012) describe why the Rasch models are appropriate for the investigation of the dimensionality of scales. The Rasch models construct a one-dimensional measurement system from ordinal data. However, more than one latent dimension will always contribute to empirical data. Multidimensionality becomes a real concern when the response patterns indicate the presence of two or more dimensions so disparate that it is no longer clear what latent dimension the Rasch dimension operationalizes.
Factor analysis is widely used in psychometrics to investigate the dimensionality of empirical data. Like other statistical analyses, it operates on interval item scores whereas the item responses are ordinal by nature. Thus results of studies using these methods are disputable. Factor analysis “is confused by ordinal variables and highly correlated factors. Rasch analysis excels at constructing linearity out of ordinality and at aiding the identification of the core construct inside a fog of collinearity” (Schumacker & Linacre, 1996, p. 470). Linacre (1998) showed that Rasch analysis followed by PCA of standardized residuals is always more effective at both constructing measures and identifying multidimensionality than standard factor analysis of the original response-level data.
A key issue in the identification of a second dimension is the choice of the critical value of the eigenvalue. Researchers have suggested various critical values. Linacre (2005), however, argues convincingly that an eigenvalue on the Rasch standardised residuals of a value of less than 2 indicates that the implied dimension in the data has less than the strength of two items and has little strength in the data.
Furthermore, with the use of infit and outfit statistics one can identify items which do not fit the models’ expectations, threatening unidimensionality. Smith (1996) emphasises that items that do not fit the model should not be automatically rejected. Instead, the reasons for their misfit should be examined before deciding whether to retain or reject them.
Research Objectives
Many researchers have accepted the arguments of Buysse at al. (1989) and used the PSQI without questioning its dimensionality. Few studies have investigated the factor structure of the scale but none has substantiated a single-factor structure and this makes using a single score on this scale questionable. Kotronoulas, Papadopoulou, Papapetrou, and Patiraki (2011) reported a 2-factor structure. Aloba et al. (2007) and Cole, Motivala, Buysse, Oxman, Levin, and Irwin (2006) reported a 3-factor structure. Furthermore, the above mentioned studies have used factor analysis techniques on the seven components and not on the 18 items from which the components were derived, and this seems to be another drawback (other than using Factor Analysis on ordinal data) of their investigations. Only one study, to the knowledge of the authors, has used Rasch measurement to validate the (revised) PSQI (Chien, Hsu, Tai, Guo, & Su, 2008) and, in that case, only 9 of the original 18 items (with a 3-point instead of a 4-point Likert scale) were used to establish a one-dimensional scale. The person reliability they reported was 0.75 and person separation 1.87.
Originally, the aim of the study was to investigate the psychometric properties of the PSQI with the use of the Rasch Rating Scale Model (Andrich, 1978; Wright & Masters, 1982) and its appropriateness for a non-clinical sample (study 1). However, results showed that the PSQI was not very appropriate for such a sample and a modified version of it was used in a second study using a 3-point instead of a 4-point Likert scale and 11 items of the original PSQI together with seven additional items, one of which was a combination of two PSQI items. Thus, it was deemed more appropriate to revise the main focus of these studies. Consequently, the aim became to investigate, with the use of the Rating Scale Model (RSM), the dimensionality and reliability of the modified instrument, whether or not the items define a theoretical linear continuum of increasing difficulty, the functioning of its Likert scale and the appropriateness of the instrument for a non-clinical sample.
Methodology
Before commencing study 1, permission was obtained from the developers of the PSQI to use the Greek version (PSQI-G). The PSQI-G was found, following their suggestion, on the MAPI Institute website. MAPI is an international company with a team of experts providing expertise in the translation and linguistic validation of patient-reported outcomes instruments for cross-cultural use and interpretation.
For the selection of the sample in study 1, a multistage stratified random sampling design was applied. In the first stage, the sampling frame was stratified into urban and rural strata by district. The households were allocated proportionally in each stratum according to the Population Census of the Statistical Service of the Republic of Cyprus which took place in 2001. The selection of households in the urban areas was implemented through a simple systematic random sample. A random digit was selected and, by using an appropriate sampling interval, the urban households for each district were selected, giving a sample of size proportional to the size of each district. The selection in rural areas was conducted in two stages: the villages of each district were the Primary Sampling Units and the households the Ultimate Sampling Units. The sample of villages was drawn with probability proportional to its size.
For the second stage, respondents were selected randomly in each household using per age and gender quotas proportional to the total population in accordance with the Population Census. In each household only one questionnaire was administered after the purpose of the study was explained in layman’s terms. The response rate amounted to 86%. Specifically, 697 households were visited and 600 questionnaires were administered.
A pilot study was conducted prior to the commencing of the survey in order to test the format, the questions and the questionnaire administration time. In total, 48 trained and experienced interviewers were employed to administer the questionnaires and clarify any ambiguities to the respondents. The fieldwork force was organized by MRC Institute Ltd, a full service research agency in Cyprus, and was monitored by the researchers. Table 1 shows the composition of the sample by gender (47% males and 53% females) and by age. Furthermore, out of the 600 participants, 415 (69.2%) lived in urban areas and the remaining 185 (30.8%) in rural areas.
Table 1
Sample Composition in Study 1: Gender by Age
Gender | Age | ||||||
---|---|---|---|---|---|---|---|
18-24 | 25-34 | 35-44 | 45-54 | 55-64 | 65+ | Total | |
Male | 42 | 56 | 57 | 48 | 39 | 39 | 281 (47%) |
Female | 45 | 59 | 67 | 60 | 39 | 49 | 319 (53%) |
Total | 87 | 115 | 124 | 108 | 78 | 88 | 600 |
Results of the data analyses indicated that, for the PSQI-G to become appropriate for a non-clinical sample, it needed modifications. Therefore, a second study was considered essential. These modifications included the removal of certain items, the addition of others and the modification of the Likert scale from 4-point to 3-point.
The sampling of the first study proved very costly and time consuming and therefore the researchers decided to select a much smaller sample in the second study, of around 200 respondents. Also, instead of the whole population of Cyprus, the target population was people living in the two largest towns of the country, Nicosia and Limassol. The procedure followed was exactly the same as for the urban households in study 1. The sample size was 203 and proportional to the populations in the two towns. Overall, 230 households were visited and 203 questionnaires were collected, giving a response rate of 88%.
Selection of the Rasch Rating Scale Model (RSM)
The Rasch RSM was selected for data analysis for the following reasons. First, the Rasch models are the only models in Item Response Theory (IRT) that accept the raw scores of the respondents to be a sufficient statistic for the estimation of their position on the variable continuum, thus maintaining the score order of students. Since raw scores are the basis for reporting results throughout all the studies, the Rasch models are consistent with practice. Second, the Rasch models involve fewer parameters and are thus easier to work with, to understand and to interpret. Third, the Rasch models give stable item estimates with smaller samples than other more general models (Thissen & Wainer, 1982). Fourth, the person measures and item calibrations have a unique ordering on a common logit scale (Bond & Fox, 2001, 2007; Wright & Masters, 1982) making it easy to see relations between them. Fifth, validity and reliability issues can be addressed through the use of the Rasch models (Smith, 2004).
Most importantly however, according to Andrich (2004), the Rasch model is based on a different philosophy from other approaches. This philosophy dictates the structure of the data including the fact that unidimensionality is a must for the measurement process. Other models are driven by a desire to model all of the characteristics observed in the data, regardless of whether they contribute to the measurement process or not. So, as Panayides, Robinson, and Tymms (2010) argue, the difference is between measurement and modelling. If the aim is to construct a good measure then the items comprising the scale should be constrained to the principles of measurement, thus the Rasch model is highly appropriate.
Selection of the Fit Statistics
The infit mean square and the outfit mean square were preferred for the present studies over a large number of fit statistics for their exploratory nature (Douglas, 1990). They can identify a wide range of potential sources of unexpected response patterns and this is an advantage. A fit statistic that focuses on a specific type of unexpectedness may not have enough power to identify other types, thus missing ‘bad’ items. Also, the infit and outfit mean squares have been used successfully to assess the fit of the Rasch models for many years (e.g. Curtis, 2004; Lamprianou, 2006; Panayides & Walker, 2012; Smith, 1990; Wright & Masters, 1982). Furthermore, these statistics are computationally simpler and stand up well in comparison with possibly more precise tests. Finally, they are utilized by most of the available software packages for Rasch calibrations (e.g. ConQuest, Winsteps, Facets, RUMM2020) and are familiar to many researchers. Therefore there is no practical reason to use anything more complicated (Smith, 1990).
Critical Values for the Fit Statistics
Wright, Linacre, Gustafson, and Martin-Lof (1994) and Bond and Fox (2001, 2007) provide a table of reasonable item mean square fit values and suggest a critical value of 1.4 for psychometric scales. Values of 1.4 indicate 40% more variability than predicted by the Rasch model. Curtis (2004) and Glas and Meijer (2003) suggest using simulated data according to a IRT model based on the estimated parameters and then determining the critical values empirically. However, Lamprianou (2006) argues that misfit is not a dichotomous ‘yes’/’no’ property but rather a matter of degree and as such it can be considered too large for one study and satisfactory for another depending on the aims of the researchers. For the purposes of the present studies, it was decided to consider items with infit or outfit greater than 1.4 (the widely used cut-off value) as ones needing re-examination before deciding to maintain or remove them from the scale, as suggested by Wright et al. (1994) and Bond and Fox (2001, 2007).
Rasch Diagnostics for the Optimal Number of Categories
A critical component influencing the measurement properties of any self-reported psychometric scale is the rating scale. Yet there is no real general agreement regarding the optimal rating scale format. Khadka, Gothwal, McAlinden, Lamoureux, and Pesudovs (2012) suggest a maximum of 5, clearly-labelled and non-overlapping categories. Wright and Linacre (1992) point out that it is the analyst’s task to extract the maximum amount of useful meaning from the responses observed by combining (or even splitting), if necessary, categories as suggested by the results of careful analysis, provided that both the statistical and substantive validity of the results is improved.
Rasch analysis provides a strong tool in the assessment of the functioning of rating scales. Linacre (2002) suggested the following guidelines for determining the optimal number of categories. First, categories with frequencies less than 10 do not provide an adequate number of observations for estimating stable threshold values. Second, the average measures of all persons in the sample who chose a particular category should increase monotonically in size as the variable increases, indicating that higher scores endorse higher categories. Third, the thresholds (or step calibrations) should also increase monotonically across the rating scale otherwise categories are considered disordered. Fourth, the range between adjacent threshold estimates should be large enough to show a distinct range on the variable (Linacre suggests at least 1.4 logits). At the same time it should not be greater than 5 logits to avoid large gaps in the variable. Step disordering and very narrow distances between thresholds “can indicate that a category represents too narrow a segment of the latent variable or corresponds to a concept that is poorly defined in the minds of the respondents” (Linacre, 2002, p. 98). Finally, outfit greater than 2 indicates more misinformation than information, thus the category introduces noise into the measurement process.
Unidimensionality
The dimensionality of the data was investigated through different studies as suggested by Linacre (1998). First, the items correlations with the total measures were calculated, followed by PCA of the standardised residuals and an examination of the item fit statistics.
Reliability Indices
The person reliability is an indication of the precision of the scale by showing how well the instrument can distinguish individuals. It can be replaced by the person separation index which ranges from zero to infinity and indicates the spread of person measures in standard error units. Finally the item reliability shows how well the items are discriminated by the sample of respondents. Wright and Masters (1982) make the point that good item separation is necessary for effective measurement.
Comparison of the Mean Measures of Distinct Groups
For validation purposes in study 2, a group of 20 adult (12 males and 8 females, aged 23 to 75) depression patients from a psychiatric private practice were added to the group of randomly selected Cypriots. The patients were considered to be poor sleepers and were used to investigate whether the modified scale would identify this distinct clinical group. Clinical evaluations of the conditions of these subjects were carried out through a complete medical history and clinical interview by their personal psychiatrist, who is one of the researchers. Taking into consideration these clinical findings, final diagnoses were made based on the DSM-IV criteria (American Psychiatric Association, 1994). The modified scale was administered to them by their psychiatrist, after the aim of the study was explained and their consent taken.
The position of the depression patients on the quality of sleep continuum was estimated using the item estimates from the non-clinical sample calibrations. Comparisons between the mean raw scores and mean Rasch measures of the two groups were then made through independent samples t-tests.
Results from Study 1: The Original PSQI-G
In the first study, the original PSQI was used. The scale was deemed not appropriate for the non-clinical sample for the following reasons:
Complicated Scoring
Many different calculations are needed in order to derive the seven component scores. Some components are described by one item and others by more. The most striking and problematic example is the score for the “Sleep Disturbances” component. The component score is calculated as the sum of responses to 9 items (with scores 0 to 3). The component score is then calculated as 0 if the sum of responses to all nine items is 0, 1 if sum = 1 – 9, 2 if sum = 10 – 18 and 3 if sum = 19 – 27. Therefore, if in any two or three items a person responds with a 3 and in other items with a 0, then the Sleep Disturbances score is 1 and that indicates no serious disturbances problems. For example, if a person wakes up during the night three or more times a week because he/she cannot breathe comfortably and because he/she experiences pains and because he/she has to go to the toilet, then his/her score on Sleep Disturbances will be very low when in fact such a person would have serious sleep disturbances.
Non-Uniform Likert Scale
Even though the 7 components do have a uniform Likert Scale (0 to 3), the individual items to which the persons respond do not. Some items have a 4-point Likert scale and some require a numerical response, such as: During the past month, what time have you usually gone to bed at night? During the past month, how long (in minutes) has it usually taken you to fall asleep each night?
Reliability Indices
The person reliability is low at 0.69 as is the person separation at 1.48. Both indices suggest a less than desirable reliability for this scale.
Validity (Unidimensionality and Item Fit)
Table 2 shows the results of PCA of the standardised residuals. There is no strong indication of a second dimension in the data (eigenvalue of 2 which explains 7.5% of the variance in the data and 11.6% of the unexplained variance) and the dimension operationalized by the Rasch model explains only 35.5% of the variance in the data. Also the ratio of variance explained by the measures to the variance explained by the first component is 4.65:1.
Table 2
PCA of the Standardised Residuals
Eigenvalue | % of total variance | % of unexplained variance | |
---|---|---|---|
Total raw variance in observations | 26.3 | 100.0% | |
Raw variance explained by measures | 9.3 | 35.5% | |
Raw variance explained by persons | 5.7 | 21.6% | |
Raw variance explained by items | 3.7 | 13.9% | |
Total Raw unexplained variance | 17.0 | 64.5% | 100.0% |
Unexplained variance in 1st component | 2.0 | 7.5% | 11.6% |
The item calibrations revealed two noteworthy facts. Six of the items were misfitting (infit and/or outfit values greater than 1.4) and these six items together with two more had point measure correlation well below 0.40 (0.27 – 0.35) and this perhaps explains why the dimension measured by the scale explains only 35.5% of the variance in the data as well as the low reliability. Description of these 6 items is given in Table 4 where the new modified scale is described.
Disordered Categories
Table 3 shows the thresholds between the categories. The problem is that the thresholds are not monotonically increasing but rather they are disordered. All other criteria were met.
Table 3
Category Thresholds
Between categories | Thresholds |
---|---|
0-1 | 0.13 |
1-2 | -0.06 |
2-3 | -0.07 |
Figure 1 shows the probability curves for the four categories and depicts the problem of disordered categories. Categories 1 and 2 never peak (i.e. they are not the most probable responses at any range of person measures).

Figure 1
Category probabilities.
The figure suggests that the distinction between category 1 “Less than once a week” and category 2 “Once or twice a week” was not clear in the minds of the respondents. This suggests that these two categories should be collapsed into one category labeled, in the opinion of the researchers, “Once or twice a week”.
Poor Targeting of Items
Figure 2 shows the item-person map. The items are targeted at persons with higher measures. The easiest items are above the mean person measure and the most difficult ones more than two standard deviations above it. The item estimates vary from -0.84 to 1.37 and this is a range of 2.21 logits, not a very wide coverage of the variable of quality of sleep. This poor targeting contributed to the low degree of reliability of the scale.
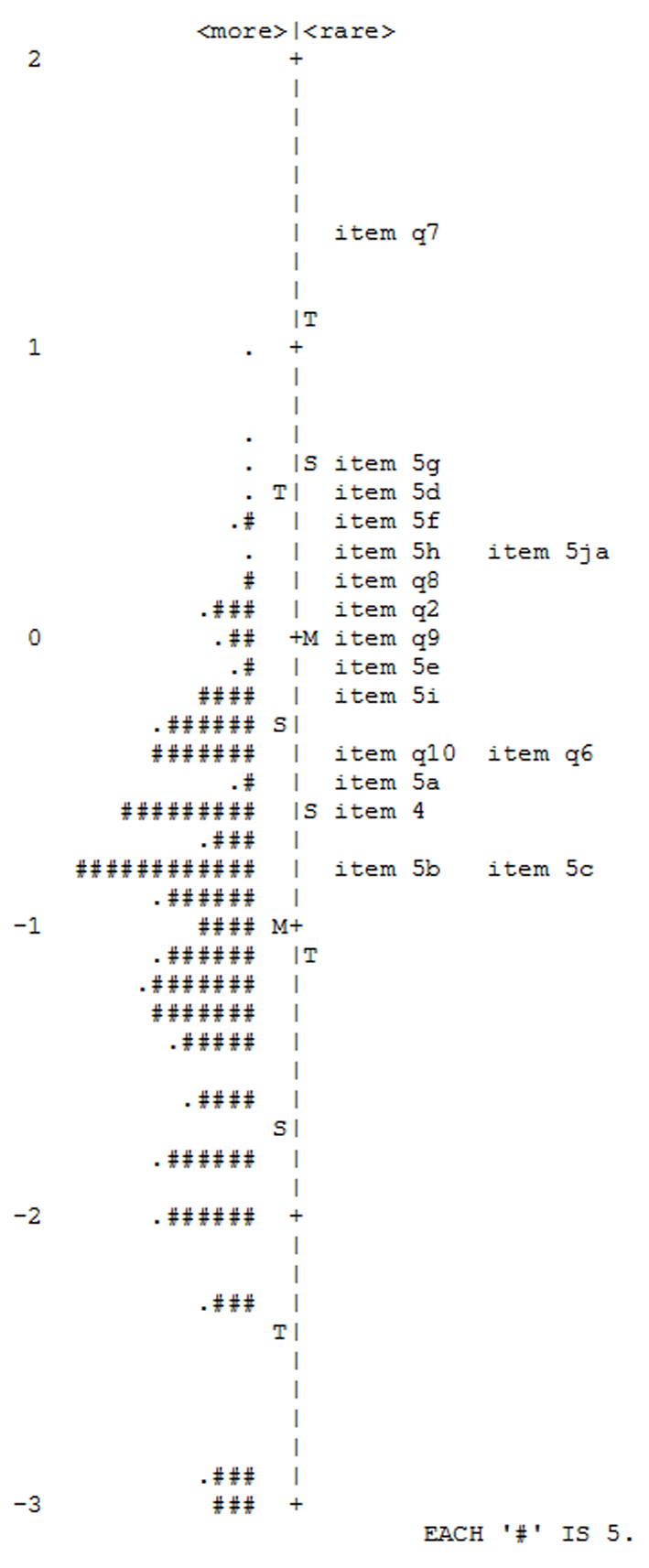
Figure 2
Person – Item map.
The Modified Scale
The following modifications, as indicated by the original analyses, were made to the PSQI-G. First the misfitting items of the PSQI-G are shown in Table 4 (all items refer to the last month):
Table 4
Misfitting Items of the PSQI-G
Item | Measure | Infit | Outfit | Description |
---|---|---|---|---|
q7 | 1.37 | 1.86 | 0.91 | How often have you taken medicine to help you sleep? |
q5e | -0.6 | 1.43 | 1.58 | How often have you had trouble sleeping because you cough or snore loudly? |
q8 | 0.21 | 1.45 | 1.58 | How often have you had trouble staying awake while driving, eating meals or engaging in social activity? |
q5ja | 0.34 | 1.52 | 1.20 | How often have you had trouble sleeping because of any other reason? |
q5g | 0.60 | 1.44 | 1.35 | How often have you had trouble sleeping because you were feeling too hot? |
q5f | 0.38 | 1.43 | 1.42 | How often have you had trouble sleeping because you were feeling too cold? |
Item q7 was the most difficult item and, being so far from the person locations, all responses greater than 0 were essentially contributing to its misfit. However, the researchers felt that taking medicine to help a person sleep is a strong indication of sleep problems and bad quality of sleep; consequently, this item was maintained into the new scale. Item 5e was also maintained because it was one of the easier items (in an attempt to improve the item targeting of the scale). Infit was marginally above 1.4 and outfit was affected by few unexpected responses. Item q8 was removed. Item 5ja was improved by describing the other possible factors (thus combining three items of the original PSQI-G into one again, in an attempt to make this specific item easier for better targeting of the items). The last two items 5g and 5f were combined into one item “During the past month, how often have you had difficulty sleeping because you were feeling too hot or too cold?” because, conceivably, it depends on the weather conditions. Finally, the item “During the past month, if you have spent eight hours in bed at night how many of those were actually spent sleeping?” replaced the three items that were used to calculate “Habitual Sleep Efficiency”. The three items were:
-
Item 1: During the past month, what time have you usually gone to bed at night?
-
Item 3: During the past month, what time have you usually gotten up in the morning?
-
Item 4: During the past month, how many hours of actual sleep did you get at night?
In order to improve the targeting of the items, the researchers added a few more items which they believed would be easier to endorse. Those were:
-
Item 12: During the past month, how often have you felt that you wanted to sleep for a few more minutes when you woke up in the morning?
-
Item 13: During the past month, how often have you felt that you have wanted to sleep for a while in the afternoon?
-
Item 14: During the past month, how often have you felt sleepy while watching television in the evening?
-
Item 15: During the past month, how often have you had difficulty getting up in the morning because you were still feeling tired?
-
Item 16: During the past month, how often have you felt sleepy straight after lunch?
The above items were taken, and adapted for this specific sample, from the Sleep Scale from the Medical Outcomes Study (RAND Corporation, 1986) and the Sleep Disorder Interactive or Sleepiness Scale (Sleep Disorder Interactive or Sleepiness Scale, n.d.).
Overall, 11 items were maintained from the original PSQI-G, two were combined, and 6 new added giving an 18-item modified scale. Also analyses suggested collapsing categories 2 and 3 and the new modified scale had a 3-point Likert scale where 0 = “Never”, 1 = “Once or twice a week” and 2 = “More than twice a week”.
Results from Study 2: The Modified Scale
First Calibration
The first analysis of the 18-item scale revealed two misfitting items. Item 14 (measure -1.55, outfit 1.51) and item 12 (measure -1.27, outfit 1.46). Both were rather easy items and had the lowest point measure correlations (0.30 and 0.39 respectively). The misfit on those items was mainly caused by quite a few unexpected 0s and 1s by those among the higher scorers. These items were removed also because the researchers felt that they are more related to tiredness during the day rather than bad quality of sleep. The final 16-item scale was analysed again (The 16-item scale is included in the appendix).
Second Calibration – Item Fit Analysis
The first analyses of the new 16-item scale revealed one slightly misfitting item, item 16 with outfit of 1.47. However, when the three worse fitting persons (with outfit > 3.0) were removed, all items fitted the Rasch model nicely. Table 5 shows the item measures, infit and outfit mean square statistics and point measure correlations. The items are displayed in measure order from the most difficult to the easiest. There seems to be a good spread of items from -1.87 to 2.56 logits, covering a range of 4.43 logits. Also, items exhibit a good fit to the model (infit and outfit values smaller than 1.4) and satisfactory point measure correlations (between 0.44 and 0.67).
Table 5
Item Statistics in Measure Order
Items | Raw score | Measure | St. error | Infit | Outfit | PtMeas. r |
---|---|---|---|---|---|---|
10 | 28 | 2.56 | 0.21 | 1.23 | 0.70 | 0.52 |
7 | 55 | 1.66 | 0.16 | 1.20 | 0.92 | 0.52 |
6 | 65 | 1.42 | 0.15 | 1.04 | 0.62 | 0.62 |
9 | 114 | 0.51 | 0.12 | 0.76 | 0.67 | 0.67 |
8 | 121 | 0.40 | 0.12 | 0.96 | 1.02 | 0.56 |
18 | 124 | 0.36 | 0.12 | 0.66 | 0.71 | 0.67 |
5 | 133 | 0.23 | 0.12 | 1.08 | 1.02 | 0.60 |
1 | 153 | -0.05 | 0.12 | 1.12 | 1.28 | 0.54 |
17 | 157 | -0.10 | 0.12 | 0.72 | 0.79 | 0.61 |
11 | 169 | -0.26 | 0.11 | 0.78 | 0.79 | 0.61 |
2 | 206 | -0.73 | 0.11 | 1.02 | 1.04 | 0.55 |
3 | 213 | -0.82 | 0.11 | 1.06 | 0.92 | 0.59 |
15 | 218 | -0.88 | 0.11 | 1.16 | 1.25 | 0.47 |
4 | 224 | -0.96 | 0.11 | 1.18 | 1.17 | 0.53 |
16 | 263 | -1.47 | 0.12 | 1.24 | 1.38 | 0.44 |
13 | 290 | -1.87 | 0.13 | 1.13 | 1.28 | 0.47 |
Mean | 158.3 | 0.00 | 0.13 | 1.02 | 0.98 | |
St. Dev. | 72.1 | 1.13 | 0.03 | 0.19 | 0.22 |
Dimensionality – PCA of Standardised Residuals
Table 6 shows the results of PCA of the standardised residuals. The variance explained by the measures is 47.2% and this is a significant improvement from the original PSQI-G (35.5%). The eigenvalue of the variance explained by the first component is 2.3 (7.5% of the unexplained variance and 14.2% of the unexplained variance, approximately the strength of two items). The two items with the highest loadings on the first component (both 0.58) were items 13 and 16. Finally, the ratio of variance explained by the measures to the variance explained by the first component is 6.2:1, which again is an improvement from 4.65:1 of the original PSQI.
Table 6
PCA of the Standardised Residuals
Eigenvalue | % of total variance | % of unexplained variance | |
---|---|---|---|
Total raw variance in observations | 30.3 | 100.0% | |
Raw variance explained by measures | 14.3 | 47.2% | |
Raw variance explained by persons | 7.5 | 24.7% | |
Raw variance explained by items | 6.8 | 22.5% | |
Total Raw unexplained variance | 16.0 | 52.8% | 100.0% |
Unexplained variance in 1st component | 2.3 | 7.5% | 14.2% |
Both items 13 and 16 had a loading of 0.58 on the first component extracted. Item 13 was “During the past month, how often have you felt that you have wanted to sleep for a while in the afternoon?” and item 16 “During the past month, how often have you felt sleepy straight after lunch?”. Both items were easy (measures of -1.47 and -1.87 respectively) and refer to tiredness in the afternoon hours and this is probably why they were distinguished by the first component.

Figure 3
Plot of person measures from two calibrations.
To investigate further whether these two items indeed measure a different component (different than quality of sleep), the researchers estimated the person measures using two different sets of items. First, the full 16-item scale and then the 14-item scale, having removed items 13 and 16 were used. The plot of the person estimates from the two calibrations is shown in Figure 3. The correlation between the two sets of person measures was 0.976.
The researchers decided to maintain the items in the scale for the following reasons. First, the points are very closely scattered to a straight line in Figure 3, as also indicated by the very high correlation between the two sets of data. This means that maintaining the two items does not affect the validity of the person measures. Second, the items have acceptable infit and outfit statistics. Third, the researchers believe that afternoon sleepiness can be considered as an indication of bad quality of sleep and those two items are the only ones which refer to it. Finally, the two items being the easiest, they improve the spread of items and coverage on the variable continuum and thus the item targeting and the reliability of the scale.
Reliability
The reliability indices are a good improvement from the original PSQI. The person reliability for the modified 16-item scale is 0.84, the person separation 2.25. Finally, the item reliability was 0.99, indicating that the items are discriminated very well by the sample of respondents.
Item Targeting
Figure 4 shows the spread of items.

Figure 4
Person – Item map.
Thirteen out of the 16 items are well targeted at a range of one standard deviation below to one standard deviation above the person mean and this range corresponds to approximately the central 70% of the person abilities. However, more importantly, the item targeting is much better than the item targeting of the original PSQI-G where there were no items below the person mean measure. In this case, six out of the 16 items are targeted at persons below the mean person ability (-0.58) starting with item 13 (-1.87) at 1.29 logits below the mean person ability.
Optimal Number of Categories
Figure 5 shows the probability curves for the three categories. It is clear that the thresholds are no longer disordered. Each category peaks for a satisfactory range of values on the variable continuum. Category 0 is the most probable for measures less than -0.68; category 1 for measures between -0.68 and 0.68, giving a range of 1.36 logits, close to the 1.4 minimum suggested by Linacre (1999); finally category 2 was the most probable for persons with measures above 0.68. All other criteria for diagnosing the optimal number of categories have been met.

Figure 5
Category probabilities.
Comparison of the Mean Scores and Mean Measures of Two Groups
The responses of the depression patients were added to the 203 respondents of the non-clinical sample and person measures were estimated for all 223 people using the item estimates from the second and final calibration. Then, both the mean person raw scores and mean person Rasch measures were compared using the independent samples t-test. Tables 7 and 8 show the results of the two t-tests. Both tests show strong evidence of difference (p < 0.001 and p = 0.004 respectively) with the depression patients having significantly higher scores and measures, thus worse quality of sleep.
Table 7
Comparisons of Raw Scores Between the Two Samples
Sample | N | Mean Score | SEM | t | df | p-value |
---|---|---|---|---|---|---|
Non-clinical | 203 | 15.73 | 0.484 | - | 22 | 0.000 |
Depression | 20 | 21.35 | 0.901 | 3.584 | 1 |
Table 8
Comparisons of Rasch Measures Between the Two Samples
Sample | N | Mean Measure | SEM | t | df | p-value |
---|---|---|---|---|---|---|
Non-clinical | 203 | -0.5840 | 0.093 | - | 22 | 0.004 |
Depression | 20 | 0.2915 | 0.161 | 2.907 | 1 |
Discussion
The Original PSQI-G
The aim of study 1 was to investigate the appropriateness of the PSQI-G for a non-clinical Cypriot sample, through an investigation of its psychometric properties. However, results suggested that it was not appropriate, for the following reasons. First, scoring of the scale was considered too complicated. Second, even though the seven components were reported on a uniform 4-point Likert scale, the 18 items that comprise those components were not. Third, the categories of the 4-point Likert scale were disordered, rendering it non-optimal. Fourth, reliability indices were lower than desired (person reliability of 0.69 and separation of 1.48). Fifth, unidimensionality of the scale was not very convincing and six items did not fit the Rasch RSM well. Finally, the item targeting was not very appropriate for this sample since the scale was designed for clinical samples and item estimates were all positioned above the mean person measure along the variable continuum and had a rather narrow spread of only 2.21 logits. This poor targeting of the items contributed to the low reliability indices and the poor fit of six of the items.
The Modified Scale
Results of the first study suggested some modifications to the original scale. Eleven items were maintained, two were combined into one and six new easier items were added in order to improve the targeting of the items. The Likert scale was changed from a 4-point to a 3-point. The researchers cannot tell whether this change from a 4-point to a 3-point rating scale was necessary as a result of possible semantic obstacles encountered through the translation, as suggested by Sechrest, Fay, and Hafeez Zaidi (1972), or due to problems with the original construction of the 4-point scale.
This modified scale was administered to a second sample of Cypriots. The first calibration revealed two misfitting items which were removed. Thus, the final form of the modified scale contains 16 items.
Psychometric Properties of the Modified Scale
All the items fitted the Rasch model very well (all items had infit and outfit mean square values below the critical value of 1.4). The items were approximately evenly spread along the linear continuum and had a spread of 4.43 logits, from -1.87 to 2.56. The item hierarchy created by the item calibrations forms a ladder with even steps of an average step length of 0.30 logits, with the easier to endorse items at the bottom and the harder to endorse at the top. Furthermore, the highly satisfactory item reliability of 0.99 indicates a good separation of the 16 items along the variable they define. It is therefore safe to conclude that indeed the items are sufficiently spread to define distinct levels along the variable and they do define a linear continuum of increasing difficulty.
The good targeting of the items, together with the good point measure correlations (all above 0.44) significantly improved the reliability of the scale (person reliability of 0.84 and separation of 2.25). The reliability indices reported in this study are much higher than those by Chien et al. (2008), who have also used Rasch analyses for a revised PSQI with only 9 items.
The unidimensionality of the scale was substantiated by the good point measure correlations, by the good fit of the items to the model and by PCA of the standardised residuals. The variance explained by the measures was above 47% of the total variance in the data, whereas the variance explained by the first component was 2.3 (7.5% of the total variance). To ensure that the first component did not describe a different dimension, the person measures were estimated twice. Once, using all 16 items and, second, by excluding the two items with the highest loadings on the first component. Both the plot of these two sets of person measures and the correlation (0.976) suggest that the two items can be maintained without threatening the validity of the person measures. Therefore, strong evidence was collected supporting the hypothesis that the scale was unidimensional.
Finally, in an attempt to assess the strength of the scale to identify distinct groups of poor sleepers, the scale was also administered to 20 clinically diagnosed depression patients. The mean raw scores and the mean measures of the two groups, the non-clinical group and the depression patients, were then compared with the use of the t-test for independent samples. Results showed that, indeed, the scale can discriminate between the two groups by identifying the poor sleepers with higher raw scores and Rasch measures.
Concluding Remarks
The present studies were, as far as the researchers have been able to ascertain, the only attempt to assess the psychometric properties of the full 18-item PSQI. Results showed that this scale was not appropriate for a non-clinical sample of Cypriots and the researchers proposed significant changes to the scale by removing a few items, adding others, and changing the 4-point to a 3-point Likert scale, thus ending with a new, modified 16-item scale.
This modified scale was shown to be unidimensional and to have a high degree of reliability. The items are well targeted for the range of person measures. They cover a wide range of the quality of sleep continuum (4.43 logits, from -1.87 to 2.56) and define a theoretical linear continuum of increasing difficulty. Furthermore, the 3-point Likert scale was shown to be optimal and the scale was found to be appropriate for a non-clinical Cypriot sample.
The aim of these studies was to devise a scale to measure the quality of sleep in non-clinical populations, primarily that of Cyprus, although the researchers believe that the scale should be suitable for similar populations. Such a scale could be used not just for research purposes in studies concerning quality of sleep (be they of an academic, medical or marketing nature), but also for initial evaluation in psychiatric practice. The scale could either be administered to an individual in order to assess his or her personal quality of sleep, or to a larger sample for research purposes. The style of the revised scale provides for simple completion as well as simple calculation of the total score, thus it is practical for all involved. Finally, given also its psychometric properties, further validations are recommended in other languages too.
Possible Limitations
Due to high costs, the sample used in study 2 for the evaluation of the psychometric properties of the new scale (N = 203) was smaller than the sample used in study 1 (N = 600) for the PSQI. This smaller sample may not give such reliable results as the first sample. However, having in mind the strengths of the Rasch models, the researchers feel that the conclusions of this study can be considered reliable.
It should be emphasised that results of the first study rendered the PSQI-G inappropriate for this specific non-clinical Cypriot sample. However, they cannot rule out the fact that, given a clinical sample for which the PSQI was designed, the psychometric properties could have been more favourable.
The professionally translated and linguistically validated PSQI-G was used in order to minimise potential inaccuracies in translation, however the possibility of problems with the “equivalence in terms of experiences and concepts” (Sechrest et al., 1972, p. 41) cannot be ruled out. It is suggested that further research with the use of this new scale be carried out on English speaking non-clinical samples for further evaluation of its psychometric properties in the original language of construction.