Introduction
The study of quality learning in motivational contexts is an important focus of inquiry in the field of educational psychology. One notable emphasis that has been researched extensively is achievement goal theory (Elliot & McGregor, 2001; Harackiewicz, Barron, Pintrich, Elliot, & Trash, 2002; Pintrich, Conley, & Kempler, 2003) and its applicability in educational settings. The bifurcation of a mastery goal orientation into an approach versus an avoidance dimension has attracted some considerable attentions of late (Elliot & Murayama, 2008; Van Yperen, Elliot, & Anseel, 2009). Situating within the framework of achievement goals other theoretical constructs, similarly, also account for the overall variance of academic learning – for example, academic engagement-disengagement (Martin & Dowson, 2009; Martin, Malmberg, & Liem, 2010; Suárez-Orozco, Pimentel, & Martin, 2009) and task values (Eccles, 2005; Hulleman, Durik, Schweigert, & Harackiewicz, 2008).
There is impetus then, from an educational psychology perspective, for us to consider the study of achievement goal orientations, academic engagement-disengagement, and task values in a secondary school setting. These psychological variables, in totality, may interact to explain quality learning and students’ performance outcomes. Our short-term longitudinal design, encompassing the use of structural equation modelling (SEM)(Bollen, 1989; Byrne, 1998; Loehlin, 2004) is relatively unique and may provide informative evidence pertaining to temporally displaced relationships. More importantly, however, a decomposition of effects may also clarify the central role of academic engagement and task values. Taking into consideration Elliot and Murayama’s (2008) study and the recent emphasis pertaining to the 2 × 2 model, we selectively focused on the potency of both mastery-approach and mastery-avoidance goal orientations in secondary school students’ learning of mathematics.
Theoretical Overview of Motivational Variables
The theoretical-conceptual model tested in the present study, as illustrated in Figure 1, amalgamates four major theoretical tenets and their contributions in the prediction of academic achievement: (i) enactive learning experiences, (ii) task values, (iii) mastery goals, and academic engagement-disengagement. The structural relations that we hypothesize are based, in part, on empirical evidence derived from previous research studies. We contend that the research focus detailed in this investigation has theoretical significance and practical relevance, especially in the contexts of secondary school teaching and learning. In this section of the article, we overview the mentioned theoretical constructs in accordance with the hypothesized relations outlined in Figure 1.
Enactive learning experiences, consonant with Bandura’s (1986, 1997) social cognitive theory, are authentically-based and may be subject to both mastery (e.g., an individual piece of assessment that seeks mastery and personal improvement) and normative criteria (e.g., am I as good as my best friend, Thomas, in Algebra?). Repeated successes in an academic subject matter, according to Bandura (1997), may assist individuals in the formation of their personal self-efficacy beliefs for academic learning (Hampton & Mason, 2003; Lent, Lopez, & Bieschke, 1991; Phan, 2012b). Self-reflection on previous performances, for instances, provide a basis for individuals to cognitively appraise their capabilities and for future improvement. Individuals who are successful tend to have heightened self-efficacy beliefs, compared to those who are unsuccessful and have learning difficulties. Consequently, on this basis, we contend that situating within the framework of social cognition (Bandura, 1986, 1997), enactive learning experiences could predict and enhance other motivation-related attributes. In the context of this research investigation, we postulate that individuals’ personal learning experiences could exert varying predictive effects on task values for learning, mastery goals, and academic engagement-disengagement.
Task values, similar to enactive learning experiences, have been featured prominently in cognition and motivation research. Task values, situating within the expectancy-value theory (Eccles, 2005; Eccles et al., 1983; Wigfield & Eccles, 2000), entail several types of subjective task values and these may explain individuals’ learning behaviours and achievement outcomes: attainment value or importance, intrinsic value, and utility value or usefulness of the task (see Wigfield & Eccles, 2000 for detail). Task values, in this analysis, query the extent to which individuals feel that an academic task at hand (e.g., solving a set of problems involving Algebra) is worth pursuing. We include task values in the present research investigation, as there is empirical evidence, derived from quantitative studies (e.g., Harackiewicz, Durik, Barron, Linnenbrink-Garcia, & Tauer, 2008; Hulleman et al., 2008; Liem, Lau, & Nie, 2008; Meece, Wigfield, & Eccles, 1990; Updegraff, Eccles, Barber, & O'Brien, 1996), to show that these are integral to classroom learning. An identification of a learning task that has utility value (e.g, "I think the course material in this class is useful for me to learn": Pintrich, Smith, Garcia, & McKeachie, 1991), say, may assist in the prediction of students’ motivation (e.g., adoption of mastery goals) and achievement outcomes beyond the immediate classroom. Tasks with intrinsic value (e.g., "I am very interested in the content area of this course": Pintrich et al., 1991), similarly, are interesting and may stimulate appreciation and intellectual curiosities, enabling individuals to interact with others in their learning (Hulleman et al., 2008).
The positive effects of task values and their potentials on individuals’ achievement goals for mastery are based on existing theoretical tenets (Eccles, 2005; Eccles et al., 1983; Wigfield & Eccles, 2000) and empirical evidence (Cole, Bergin, & Whittaker, 2008; Liem et al., 2008; Phan, in press). We focus on mastery goals, given there is recent emphasis for the cultivation of mastery and deep learning (Phan, 2013b). Rather than to seek alternatives and strategies that could resolve deficits and maladaptive practices (e.g., an individual seeking to adopt a work-avoidance goal), it has been suggested that educators use positive psychology theories to stimulate and encourage educational and adaptive outcomes. We emphasize, in this case, the potency of mastery goals and academic engagement for learning.
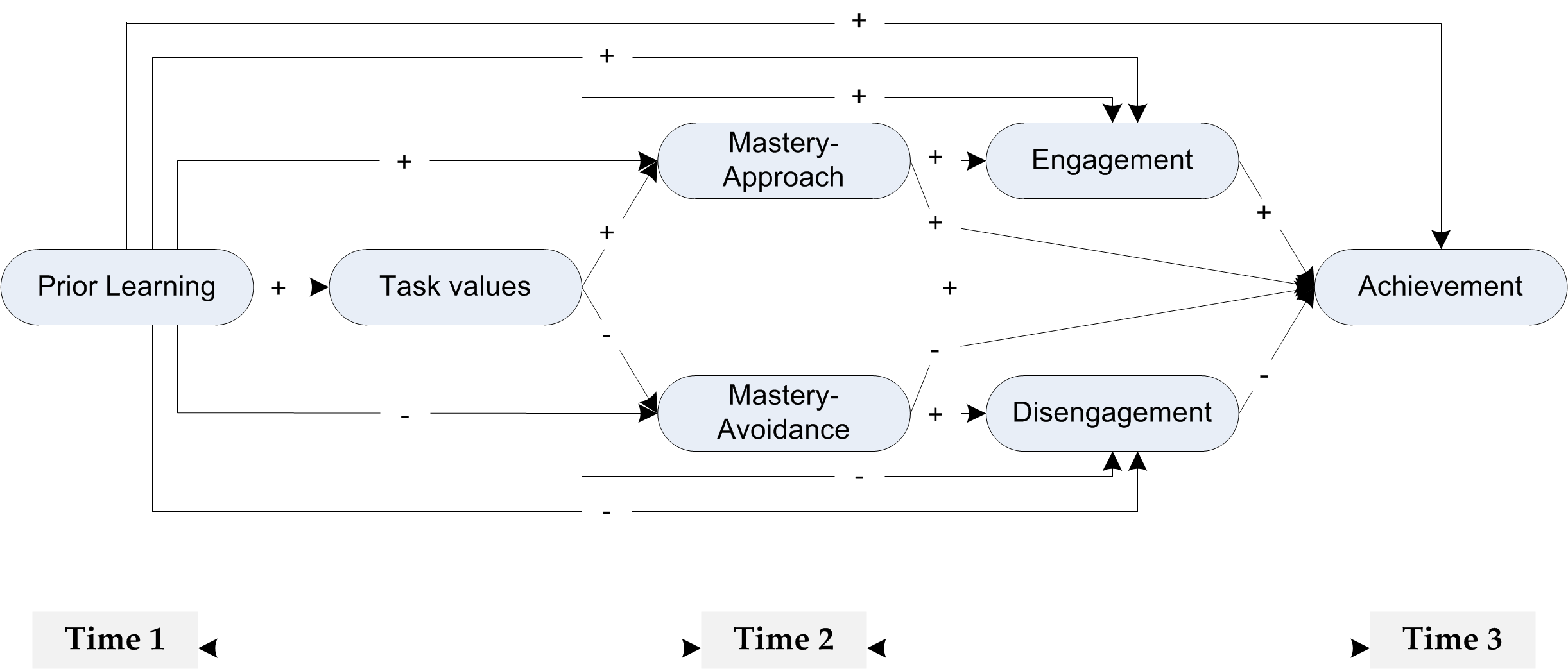
Figure 1
A conceptual framework of mastery goals, task value, and academic engagement-disengagement.
Note. ‘+’ denotes a positive relationship between the variables (e.g., the positive effect of task value on a mastery-approach), whereas ‘-’ denotes a negative relation (e.g., the positive effect of task value on a mastery-avoidance).
We focus on both mastery-approach and mastery-avoidance goals (Elliot & McGregor, 2001; Elliot & Murayama, 2008) for academic learning. These two types of mastery goals are part of the achievement goal theoretical tenets (Ames & Archer, 1988; Harackiewicz, Barron, Pintrich, et al., 2002; Nicholls, Cheung, Lauer, & Patashnick, 1989). As a definition, achievement goals are defined as “competence-relevant aims that individuals strive for in achievement settings“ (Pekrun, Elliot, & Maier, 2009, p. 115). Achievement goals, in educational contexts, enable educators and researchers to explain individuals’ patterns in cognition, motivation, thoughts, and behaviours (e.g., why does an individual adopt a deep cognitive approach to learning).
In the field of educational psychology, educators and researchers have used achievement goal theoretical tenets to explain and predict individuals’ motivation and quality learning outcomes. In the earlier years, the dichotomous model was prominent and detailed two major achievement goal types: mastery, or task-involvement (e.g., emphasizes the development of competence), versus performance, or ego-involvement (e.g., demonstrate outward competence)(Ames & Archer, 1988; Nicholls, 1984). This mastery-performance differentiation, according to researchers, is rather limited and simplistic in nature. Individuals’ motivational patterns and their approaches to learning, in this analysis, cannot simply be explained by this mastery-performance distinction, alone. For example, in earlier research studies, evidence yielded showed an inconclusive pattern for the adoption of performance goals: some researchers found negative effects for performance goals (Elliot & Church, 1997; Elliott & Dweck, 1988), whereas others full a null effect (Elliot & Harackiewicz, 1996; Harackiewicz & Elliot, 1993).
Elliot, Harackiewicz, and others, on this basis, revised the two-factor model of achievement goals to include a trichotomous model (Elliot & Church, 1997; Elliot & Harackiewicz, 1996). This three-factor model stipulates a bifurcation in the performance categorization, wherein two distinctive components are recognized: approach and avoidance (Elliot & Church, 1997; Elliot & Harackiewicz, 1996). The approach orientation (i.e., performance-approach) focuses on individuals acquiring positive possibilities (e.g., attaining competence and demonstrating superiority)(Van Yperen et al., 2009), whereas the avoidance orientation (i.e., performance-avoidance) focuses on individuals avoiding negative possibilities (e.g., failure or looking incompetent, normatively). The trichotomous model, in totality, suggests that there are three major types of achievement goals: mastery, performance-approach, and performance-avoidance. An examination of the literature (Alkharusi, 2010; Harackiewicz, Barron, Pintrich, et al., 2002; Senko, Hulleman, & Harackiewicz, 2011) indicates that there is a plethora of research, which has demonstrate the validity and utility of this trichotomous framework (Elliot, McGregor, & Gable, 1999; Fenollar, Román, & Cuestas, 2007; Liem et al., 2008; Phan, 2010).
Similar to the dichotomous model of achievement goals (Ames & Archer, 1988; Nicholls, 1984), however, the trichotomous model was limited in its explanation of individuals’ motivation in terms of competence-based strivings. Researchers, on this basis, proposed a bifurcation of mastery goals (i.e., mastery-approach and mastery-avoidance), resulting in a 2 × 2 framework (Elliot, 1999; Elliot & McGregor, 2001; Harackiewicz, Barron, Pintrich, et al., 2002; Pintrich, 2000). Overall, this model suggests four major goal types: (i) a mastery-approach orientation where individuals seek to achieve mastery or improvement, (ii) a mastery-avoidance orientation where individuals seek to avoid failing achievement of a task mastery, (iii) a performance-approach where the main focus is for individuals to accomplish and outperform others, and (iv) a performance-avoidance where one seeks to avoid doing worst than others in given tasks.
The 2 × 2 model, to date, has received less research attention than the trichotomous model. There has been some research within the past decade from scholars seeking to clarify the validity of the four types of achievement goals (Cury, Elliot, Da Fonseca, & Moller, 2006; e.g., Elliot & McGregor, 2001; Elliot & Murayama, 2008; Elliot & Thrash, 2010; Phan, 2013c). The last few years have seen researchers exploring the two types of mastery goals, in particular (Ciani & Sheldon, 2010; Madjar, Kaplan, & Weinstock, 2011; Phan, 2014; Van Yperen et al., 2009). One notable avenue of inquiry has, in this case, entailed the legitimacy and appropriateness of the 2 × 2 model, and whether mastery-avoidance goals actually exist in educational settings (Phan, 2014; Sideridis & Mouratidis, 2008). Our examination, in this analysis, may produce fruitful information and evidence pertaining, specifically, to the two types of mastery goals for academic learning.
It is interesting to note that there has been mixed evidence in relation to the relationship between goal orientations and task values, depending on one’s own conceptualization of goals. Goals, in the context the expectancy-value theory (Eccles et al., 1983; Wigfield & Eccles, 2000), differ in scope and content from the goals that are conceptualized in the achievement goal theory (e.g., Ames, 1992; Elliot, 1999; Harackiewicz, Barron, Pintrich, et al., 2002; Pintrich et al., 2003). Notably, according to Wigfield (1994), the emphasis in goals from an expectancy-value perspective connotes broader objectives and purposes for students (e.g., a student considering a particular career choice option). Goals, in the spectrum of achievement goal theory, emphasize immediacy and narrow aims and objectives (e.g., "My aim is to completely master the material presented in this class": Elliot & Murayama, 2008). As previous research studies have reported (e.g., Greene, Miller, Crowson, Duke, & Akey, 2004; Harackiewicz et al., 2008; Liem et al., 2008; Simons, Dewitte, & Lens, 2004), individuals’ task values (e.g., task interest) relate closely to various types of achievement goals. Harackiewicz et al.’s (2008) longitudinal study, for example, yielded consistent evidence, attesting to the impact of interest on various types of achievement goals (e.g., mastery).
The contributing effects of enactive learning experiences, task values, and mastery goals on academic learning and achievement-related outcomes have been attested from existing research studies. One notable achievement-related outcome, which has featured prominently in the empirical literature, is one’s engagement towards schooling and academic learning. Academic engagement and disengagement, similar to achievement goals and task values, has been researched extensively and is integral to our understanding of individuals’ learning patterns, behaviours, and motivation (Artino & Stephens, 2009; Fredricks, Blumenfeld, & Paris, 2004; Liem et al., 2008; Martin & Dowson, 2009; Willms, 2003). Academic engagement is multifaceted and diverse in its scope and coverage. As a definition, according to Willms (2003), student engagement refers to “the extent to which students identify with and value schooling outcomes, and participate in academic and non-academic school activities” (Willms, 2003, p. 8). Considering it as a multifaceted construct, encompassing the behavioural (e.g., the idea of participation in academic and/or extracurricular activities), emotional (e.g., positive and negative reactions to teachers, classmates, and school), and cognitive (e.g., the idea of investment, such as effort expenditure to comprehend complex ideas) components (Fredricks et al., 2004), Willms’ (2003) theoretical view may be broad to include students’ attitudes towards schooling and their participation in learning and school activities. Disengagement or the term “disengaged from school” (Willms, 2003), in contrast, characterizes those students who withdraw from school activities and feel they do not belong at school.
There is moderate research in the motivation literature, detailing the importance of engagement as a predictor of schooling outcomes (e.g., academic performance). Research emphasis pertaining to academic engagement is rather interesting, inferring that this theoretical construct functions, in tandem with other attributes (e.g., school culture), as a deposition towards quality learning in educational settings (Willms, 2003). From a maladaptive perspective, in contrast, there is also the inquiry that emphasizes the long-term detrimental effects of disengagement (Henry, 2010; Henry, Knight, & Thornberry, 2012; Liem et al., 2008; Offord & Waters, 1983; Salamonson, Andrew, & Everett, 2009). Offord and Waters (1983) found that, for example, adolescents who manifest and have behavioural problems (e.g., participating in anti-social and criminal activities) tend to experience disconnectedness and disengagement from school. Henry et al.’s (2012) research with youths reported a similar pattern in findings, wherein school disengagement relates to student dropout and other serious behavioural problems.
The Current Study: Enactive Learning Experiences, Task Value, Mastery Goals, and Academic Engagement/Disengagement
Integrating multiple theoretical tenets into the study of secondary school students’ motivation and learning has empirical merits and practical relevance for educators and researchers, alike. Examination of the empirical literature, described in the preceding sections, indicates that both mastery goals could function as antecedents of academic engagement and disengagement. These variables may also, in turn, operate dynamically as proximal and distal predictors of quality and achievement outcomes. In the current study, consistent with Bandura’s (1986, 1997) social cognitive theory and existing evidence (Britner & Pajares, 2006; Hampton, 1998; Lent et al., 1991; Phan, 2012a), we designed a theoretical-conceptual model that hypothesized enactive learning experiences as a focal point of origin. Enactive learning experiences would, in turn, exert varying effects on three four outcomes: task values for learning, mastery goals, academic engagement-disengagement, and achievement in mathematics learning.
The Impact of Enactive Learning Experiences
Enriched learning experiences, situated within the framework of social cognition (Bandura, 1986, 1997), form the basis of intrinsic motivation, mastery goals, students’ academic engagement and their achievement outcomes. Personal accomplishments in a subject matter, as we alluded previously, form the basis of intrinsic motivation, and may exert positive effects on task values, mastery goals, academic engagement, and achievement outcome. Task values, in accordance with the expectancy-value theoretical model (Eccles et al., 1983; Wigfield & Eccles, 2000), as indicated from existing evidence (Cole et al., 2008; Liem et al., 2008; Phan, in press), are expected to influence students’ adoption of mastery-approach goals. For example, we expect to find that perceived usefulness or intrinsic value of a particular learning activity to enhance students’ striving mastery and improvement of personal competence. Task value, in totality, in this analysis, may reflect a sense of proactivity towards schooling, and instil positive beliefs that academic learning is worthwhile for consideration and pursuing.
Mastery Goals and Academic Engagement-Disengagement
The impact of mastery goals, from 2 × 2 theoretical perspective (Elliot, 1999; Elliot & McGregor, 2001), has relevance and educational importance in terms of applied practices. The positive effect of mastery-approach goals, in particular, has been noted in previous research (Elliot & Murayama, 2008; Phan, 2013c; Sins, van Joolingen, Savelsbergh, & van Hout-Wolters, 2008), and on this basis, we contend a similar pattern in results would extend to students’ academic engagement and achievement outcome. We posit that a mastery-approach goal orientation, for instance, would relate closely to academic engagement in schooling. Willingness to seek mastery and improvement in key learning concepts of a subject matter may, of course, facilitate and motivate students to engage more proactively with the academic-social milieu for assistance. A mastery-avoidance goal, in contrast, may not necessarily translate to positive achievement-related outcomes. This type of mastery goals, as we mentioned previously, is still in its infancy in terms of research development and very little is known about its characteristics (Ciani & Sheldon, 2010; Madjar et al., 2011; Sideridis & Mouratidis, 2008; Van Yperen et al., 2009). We query, for instance, whether the avoidance component of the valence dimension could, in fact, result in negative outcomes and maladaptive practices? Does a mastery-avoidance goal orientation facilitate students to disengage and withdraw from school, altogether? Evidence ascertained, say, between a mastery-avoidance goal and academic disengagement would inform us of the potential detrimental consequences of this goal type.
Academic engagement-disengagement in educational settings is an important notion for consideration, especially in terms of national policy development, workforce and social welfare planning. Academic engagement-disengagement, as research has shown, entails both positive and negative consequences for students (Fredricks et al., 2004; Henry et al., 2012; Salamonson et al., 2009). Disengagement, for example, has been shown to result in detrimental outcomes, such as student withdrawal, participation in antisocial behaviours, etc. We need to consider, in contrast, the importance of academic engagement, given this facet has been noted to enhance student learning in achievement contexts. How do we foster a climate of school belonging, whereby students may feel inclined and motivated to engage in schooling and partake in academic and non-extracurricular activities? What preventive measures could we incorporate to ensure students do not experience a sense of isolation, stigmatization, etc.? This avenue of inquiry, from our point of view, has implications for educators and school, in general.
Enhancement of Academic Achievement
Finally, we need to consider the enhancement of learning in achievement contexts. In this investigation, as depicted in Figure 1, we amalgamate different strands of research inquiries and posit four possible predictors of achievement outcome: enactive learning experience, task values, mastery goals, and academic engagement-disengagement. In many cases, for example, there is consistent evidence to support the hypothesized structural paths between the proposed predictors and academic achievement (e.g., the negative consequences of academic disengagement, such as early dropout and juvenile deliquency: Henry, 2010; Henry et al., 2012; Moretti, 2005; Rouse, 2005). What is preliminary and unclear at present, however, is the impact of the two types of mastery goals on learning and academic achievement outcomes. Comparing to the trichotomous model of achievement goals, research pertaining to the 2 × 2 model has been modest with some evidence attesting to the effects of both mastery-approach and mastery-avoidance goals. Consequently, our examination is in accordance with the 2 × 2 achievement goal model (Elliot, 1999; Elliot & McGregor, 2001; Elliot & Murayama, 2008; Harackiewicz, Barron, Pintrich, et al., 2002), may provide additional support and clarification into the varying patterns of predictive and explanatory effects for both mastery-approach and mastery-avoidance goal orientations.
In summation, in accordance with Figure 1, the focus of our research investigation details four major research hypotheses:
Hypothesis 1. It is hypothesized that task values, a mastery-approach goal orientation, and academic engagement would exert positive predictive effects on academic achievement in mathematics.
Hypothesis 2. It is hypothesized that both mastery-avoidance goal orientation and academic disengagement would exert negative predictive effects on academic achievement in mathematics.
Hypothesis 3. It is hypothesized that both mastery-approach and mastery-avoidance goal orientations would exert positive and negative predictive effects on academic engagement and disengagement, respectively.
Hypothesis 4. It is hypothesized that task values would exert positive predictive effects on a mastery-approach goal orientation and academic engagement, and negative predictive effects on a mastery-avoidance goal orientation and academic disengagement.
In Totality: An Integrated Framework
The significance of the present study then, as outlined in Figure 1, entails a longitudinal examination of interrelations between different motivational and cognitive processes of learning. We focus on the bifurcation of mastery goals, an important focus in educational research that requires clarity and validation. Furthermore, contributing to the study of student learning in achievement contexts, we also included both engagement and disengagement as potential adaptive and maladaptive outcomes of academic learning. This inclusion, we feel, is pivotal to the understanding of learning and achievement within a larger system of academia. The stipulation of academic engagement, for example, may provide evidence that spans beyond the individualistic trait perspective (Phan, 2014).
Methods
Sample
Two hundred and ninety-two 12th grade students took part in this short-term longitudinal study, which was funded and approved by our university. The data, from three government schools in Western Sydney, was collected in both 2012 and 2013. Western Sydney, overall, is multicultural and the three schools involved were average in sociocultural status and diverse in ethnic and cultural compositionsi. Many students from these three schools, first, second and third generation Australians would be of European, Asian, and Middle Eastern backgrounds. Given the final two years of secondary schooling in Australia are crucial, logistically, we had very limited, if any, opportunities to stipulate our own preference in time points in data collection. With the structured busy schedules of 12th grade classes, in particular, we managed to complete the questionnaires in less than 40 minutes, including instructions, administration, and clarification of questions that some students had for us. Due to missing data, a final sample of 262 (139 boys and 123 girls) was included in the subsequent statistical analyses.
The three time points of data collection in this study are as follows: (i) Time 1 in Early-December, 2012, with the collection of students’ 11th grade results in mathematics, (ii) Time 2 in the first week of February, 2013, with the administration of the questionnaires for task values, mastery goals, and academic engagement-disengagement, and (iii) Time 3 in June, with the collection of students’ 12th grade school term 1 results in mathematics. This short-term longitudinal examination, with multiple time points in data collection, is advantageous, theoretically, and may provide fruitful information into the temporally displaced influences of psychological variables. There has been precedence, more recently, for researchers to explore and study cognitive and motivational variables in educational settings within a longitudinal framework (Bong, 2001; Durik, Lovejoy, & Johnson, 2009; Harackiewicz, Barron, Tauer, & Elliot, 2002; Harackiewicz et al., 2008; Phan, 2011a; Wong & Watkins, 1998). The educational course of one’s learning does not occur instantaneously, but rather progresses over a period of time. In the secondary schooling system, for example, 11th and 12th grades are predominantly the important years, prior to university studies. An undergraduate Liberal Arts degree, similarly, may take three to four years to complete. In this sense, from an educational perspective, an examination of temporally displaced relations between the variables (e.g., a mastery goal orientation at Time 2 → academic achievement at Time 3) would provide an in-depth understanding of the extent of potency of the mastery-approach and mastery-avoidance goals, in particular.
We administered three Likert-scale inventories, as explained below, in classes with the assistance of a doctoral student. University protocols, including ethical procedures were explained and followed. For example, apart from the notion of anonymity, we also explained to the students that their participation was voluntary and why there their results for mathematics were needed.
Instruments
One subject discipline that is important is mathematics, and many secondary school students in Australia undertake this subject with a view to use it as a pre-requisite for university entry into various undergraduate courses. Consequently, where appropriate, we asked the participants to situate their considerations and responses within the contexts of mathematics learning. Cronbach’s alpha values for our study were: .92 and .95 for the Mastery-approach and Mastery-avoidance subscales, respectively, .88 for the Task value subscale, and .95 and .90 for the Engagement and Disengagement subscales, respectivelyii.
Enactive Learning Experience
We used students’ previous 11th grade yearly results for mathematics as an index of prior mathematics achievement. This result index, for each participant, constituted in-class, periodic assessment tasks (e.g., a group project) and end-of-term examinations, and consequently, from our point of view, reflected enactive learning experiences. A number of researchers have used this methodological approach in self-efficacy studies (e.g., Liem et al., 2008; Matsui, Matsui, & Ohnishi, 1990; Phan, 2012a) instead of self-reporting responses from Likert-scale questionnaires (e.g., "I got a high grade in last year's math class": Lent et al., 1991). Student self-reporting, commonly used in previous research (Hampton, 1998; Lent et al., 1991; Usher & Pajares, 2009), has been reasoned to provide inaccurate information about one’s own judgments and reflections of mastery experiences – for example, noting down the receiving of good academic grades when, in fact, this is not true (Phan, 2011b).
Task Values
We used the task value subscale of the Motivated Strategies for Learning Questionnaire (MLSQ)(Pintrich, Smith, Garcia, & McKeachie, 1993) to measure task values. The subscale, containing six items measured on a 7-point rating scale (Strongly disagree) to 7 (Strongly agree)), describes values pertaining to interest (e.g., “I am very interested in the content area of this subject, mathematics”), perceived usefulness (e.g., “I think I will be able to use what I learn in this subject, mathematics, in other subjects”), and importance (e.g., “It is important for me to learn the subject material in this class”).
Mastery Goal Orientations
We used the two mastery goal subscales of the Revised Achievement Goal Questionnaire (ACG-R)(Elliot & Murayama, 2008) to measure students’ mastery goal orientations. Each subscale, containing three items each and measured on a 7-point rating scale (1 (Strongly disagree) to 7 (Strongly agree)), was modified slightly to fit in with the contexts of mathematics learning for secondary school students. The sample items included, for example: “My aim is to completely master the material presented in this class” (Mastery-Approach item) and “My aim is to avoid learning less than I possibly could” (Mastery-Avoidance item).
Academic Engagement and Disengagement
There are a number of inventories, which measure and assess students’ academic engagement (Martin, 2001; Martin & Marsh, 2005; Schaufeli, Salanova, González-Romá, & Bakker, 2002). We chose to use Schaufeli et al.’s (2002) Engagement Scales, Student Version, as these have been found to demonstrate good construct validity, relevance, and applicability to classroom learning. The 17 items, answered on a 7-point rating scale (1 (Strongly disagree) to 7 (Strongly agree)), defined three distinct dimensions: Vigor (e.g., “When I get up in the morning, I feel like going to class”), Dedication (e.g., “To me, my studies are challenging”), and Absorption (e.g., “When I am studying, I forget everything around me”). Similar to the stipulation and procedure of personal self-efficacy (Bandura, 1997; Pajares, 1996), we asked the participants to rate their judgments for these items within the context of mathematics learning. For example, in relation to the absorption dimension, it would be, say: …. When I am studying [the subject mathematics], I forget everything around me.
Significantly, from our point of view, the inclusion of disengagement may also provide theoretical insights into its characteristics compared to academic engagement. Can it be inferred that disengagement, as a negative valence, is simply a ‘reverse’ of academic engagement, as a positive valence? Some researchers (e.g., Salamonson et al., 2009) have, for example, made reference to these two theoretical terms casually and interchangeably without consideration of differences or similarities. Rather than inferring logically that disengagement is a reverse of academic engagement, we reasoned that an actual measure would be beneficial, theoretically and methodologically. We used two items of Martin and Marsh’s (2005) research (e.g., “I often feel like giving up in this subject, mathematics”) and adapted nine items from Artino and Stephens’ (2009) version of Wolters’ (2004)(e.g., “I often find excuses for not starting the work for this mathematics class”) research to measure students’ academic disengagement. Of the 11 items, which were rated on a 7-point Likert scale (1 (Completely disagree) to 7 (Completely agree), two items were worded positively (e.g., “I look forward to learning more about this subject, mathematics, in the future”) and nine negatively (e.g., “I often find excuses for not starting the work for this mathematics class”).
Academic Achievement in Mathematics Learning
We defined academic achievement in this investigation as a measure of two indexes: (i) Course mark that is made up of periodic quizzes and other assessment tasks, and (ii) End-of-term examination. These two indexes, similar to our previous mentioning, reflect a measure of enactive learning experiences for mathematics. Ideally, from the perspective of constructive alignment (Biggs, 1999), we would have preferred to use a formal test and other in-class assessment tasks to gauge into participants’ learning and achievements in mathematics. Having said this, however, the upper secondary school years in Australia are relatively crucial (e.g., preparing for entry into university), and consequently, we did not have the flexibility to implement such course of action.
Results
We decide to use structural equation modelling (SEM) procedures (Byrne, 1998; Kline, 2011; Loehlin, 2004), aided by the SPSS AMOS 20 software package, to test and validate the hypothesized relations mentioned in the preceding sections. This statistical technique, in contrast to other multivariate approaches for its emphasis on both latent factors and measured indicators, has been used in previous research to explore the relations between personal self-efficacy and other related motivational-related variables (e.g., Fenollar et al., 2007; Liem et al., 2008; Phan, 2010; Sins et al., 2008). SEM is more advantageous at it assumes that measured indicators have errors (i.e., E ≠ 0), and that we can assess and evaluate both measurement and structural relationships simultaneously. Furthermore, with the availability of modification fit index (MI) and goodness-of-fit index values, SEM allows researchers to propose and test competing a priori models for acceptance. In relation to the latter, there are a number of goodness-of-fit index values available, but for this investigation, similar to previous studies, we chose to use the following: the ratio between chi-square statistics and degree of freedom (χ2/dƒ)(χ2/dƒ value ≤ 5.00), Comparative Fit Index (CFI)(CFI value ≥ .90), the Non-normed Fit Index (NNFI)(NNFI value ≥ .90), and the Root Mean Square Error of Approximation (RMSEA)(RMSEA value ≤ .080).
Structural Relations
The descriptive statistics, involving the means and standard deviations for the total sample and individual groups (boys versus girls) are presented in Table 1. In our initial data screening, we note that the data was normally distributed with kurtosis and skewness values for the variables falling within the +/- 2.00 (Byrne, 1998). SEM procedures stipulate, in particular, the forming of aggregate scores to represent multiple measured indicators for each latent factor (Byrne, 1998; Marsh & Yeung, 1997). For each latent factor, for example, there is a requirement of more than one measured indicator. The use of aggregated scores, recommended and used in previous motivational and social sciences research (e.g., Lent, Lopez, Brown, & Gore, 1996; Marsh & Yeung, 1997; Vispoel, 1995), is advantageous as these are more reliable and valid, decrease the effects of idiosyncrasies associated with individualized items, and reduce the computer resources required for complex analyses (Marsh & Yeung, 1997). On this basis, for the statistical analyses reported in this study, we used the procedures recommended by Marsh and Yeung (1997) to form our parcel items. There are, in total, five latent factors (sequencing from left to right in Figure 1: task value, mastery-approach and mastery-avoidance goal orientations, and academic disengagement and engagement), and 15 parcel items, with each latent factor defined by three items. Furthermore, we treated both prior learning experience and achievement in mathematics as measured variables. Overall then, there are 17 measured indicators used in the subsequent structural equation analyses.
Table 1
Descriptive Statistics of Means and Standard Deviations.
Instruments | Means
|
||
---|---|---|---|
Boys | Girls | Total sample | |
Task values | 2.285 (1.012) | 2.328 (1.052) | 2.305 (1.029) |
Mastery-approach | 5.257 (.903) | 5.427 (.804) | 5.337 (.861) |
Mastery-avoidance | 5.478 (1.466) | 5.630 (1.254) | 5.550 (1.370) |
Academic engagement | 5.395 (1.468) | 5.304 (1.521) | 5.352 (1.491) |
Academic disengagement | 2.250 (1.005) | 2.307 (1.043) | 2.277 (1.022) |
Prior learning experience | 62.573 (14.372) | 61.866 (14.287) | 62.241 (14.235) |
Achievement in mathematics | 68.885 (7.153) | 69.648 (6.307) | 69.243 (6.767) |
Note. Standard deviations are shown in brackets.
Our statistical testing of the hypothesized model, Model M0, as illustrated in Figure 1, included the freeing of 17 structural paths: (i) prior learning experience to task value, mastery-approach and mastery-avoidance goals, academic engagement and disengagement, and academic achievement, (ii) task value to mastery-approach and mastery-avoidance goals, academic engagement and disengagement, and academic achievement, (iii) a mastery-approach goal to academic engagement and academic achievement, (iv) a mastery-avoidance goal to academic disengagement and academic achievement, and (v) academic engagement and disengagement to academic achievement. The goodness-of-fit values for Model M0 indicated an excellent model fit, as reflected by the following: NNFI = .990, CFI = .998, and RMSEA = .024.
Progressing on from the hypothesized model, we tested four competing a posteriori alternatives, as shown in Table 2. Model M1, Model M2, and Model M3 are an extension of Model M0 with the exception of the following: (i) regression of a mastery-approach goal onto academic disengagement, (ii) regression of mastery-avoidance goal onto academic engagement, and (iii) regression of a mastery-approach goal onto academic disengagement, and a mastery-avoidance goal onto academic engagement. The goodness-of-fit indexes for the three models showed excellent model fits, with CFI, NNFI, and RMSEA values satisfying the benchmark requirements (i.e., CFI and NNFI > .90, RMSEA < .08). Chi-square difference tests indicated, however, that there was no statistical difference between Model M0 and Model M1 (∆χ2 = .357, p > .05), between Model M0 and Model M2 (∆χ2 = 2.053, p > .05), and between Model M0 and Model M3 (∆χ2 = 2.410, p > .05). The principles of parsimony would recommend the acceptance of a more simplistic model as a final solution (e.g., Model M3). Having said this, though, we decided to retain Model M0, as the proposed a posteriori structural paths from a mastery-approach goal to academic disengagement and from a mastery-avoidance goal to academic engagement were statistically nonsignificant.
Model M4, in contrast, specified the fixing of the structural paths from task value to a mastery-avoidance goal and academic disengagement. This a posteriori statistical testing was based on the rationale that task values (e.g., intrinsic value of a learning task), as positive motivational beliefs, are dissonant with maladaptive practices such as an adoption of a mastery-avoidance goal orientation. The goodness-of-fit index values for Model M4 (e.g., CFI and NNFI < .90), below the optimal values, showed a poor model fit. Furthermore, a chi-square difference test indicated statistical significance at the p < .001, suggesting the acceptance of the less constrained model, Model M0. A summary of the goodness-of-fit index values for the four models tested is presented in Table 2.
On this basis, with a preference for Model M0, we depict the final solutions in structural relations in Figure 2. For clarity, we have omitted the non-statistical significant paths. All standardized paths are significant at the p < .05 and p < .01. In addition to Figure 2, a decomposition in direct and indirect effects is shown in Table 3. In total, there are six statistical significant structural paths. Prior learning experience exerted a negative effect on a mastery-approach goal (β = -.18, p < .01), whereas task values exerted a positive effect on academic disengagement (β = .82, p < .001). Both mastery-approach and mastery-avoidance goals influenced academic engagement (β = .13, p < .05) and disengagement (β = -.08, p < .05), respectively. Achievement in mathematics is also influenced by both mastery-approach (β = .13, p < .05) and mastery-avoidance (β = .13, p < .05) goal orientations. Finally, for indirect relationships, only two small effects were observed: the indirect negative effect of prior learning on academic engagement (β = -.023, p < .05) and achievement in mathematics (β = -.022, p < .05), via a mastery-approach goal.
Table 2
Goodness-of-Fit Index Values
Description | χ2/ dƒ | Comparison | ∆χ2 | NNFI | CFI | RMSEA | |
---|---|---|---|---|---|---|---|
Model M0 | |||||||
Hypothesized a priori model with the freeing of 17 structural paths. | 1.151 | *** | - | - | .990 | .998 | .024 |
Model M1 | |||||||
A posteriori analysis of Model M0 with the freeing of structural paths from:
|
1.349 | *** | M0 – M1 | .357 | .976 | .996 | .037 |
Model M2 | |||||||
A posteriori analysis of Model M0 with the freeing of structural paths from:
|
.926 | *** | M0 – M2 | 2.053 | 1.00 | 1.00 | .001 |
Model M3 | |||||||
A posteriori analysis of Model M0 with the freeing of structural paths from:
|
1.115 | *** | M0 – M3 | 2.410 | .992 | .999 | .021 |
Model M4 | |||||||
A posteriori analysis of Model M0 with the fixing of structural paths from:
|
20.979 | M3 – M0 | 141.096 | .350 | .550 | .277 |
***p < .001.
A Posteriori Analysis: Gender Differences
We performed one-way between-groups multivariate analysis of variance to investigate gender differences between the variables. Seven dependent variables were used: prior learning experience, task value, mastery-approach and mastery-avoidance goal orientations, academic engagement and disengagement, and achievement in mathematics. The independent variable was gender. Preliminary assumption testing was conducted to check for normality, linearity, univariate and multivariate outliers, homogeneity of variance-covariance matrices, and multicollinearity (Pallant, 2007; Tabachnick & Fidell, 2007). We note that no serious violations were made. The results, in general, indicated no statistical significant difference between boys and girls on the combined dependent variables: F(7, 254) = .647, p = .717; Wilks’ Lambda = .982, partial eta squared = .018.
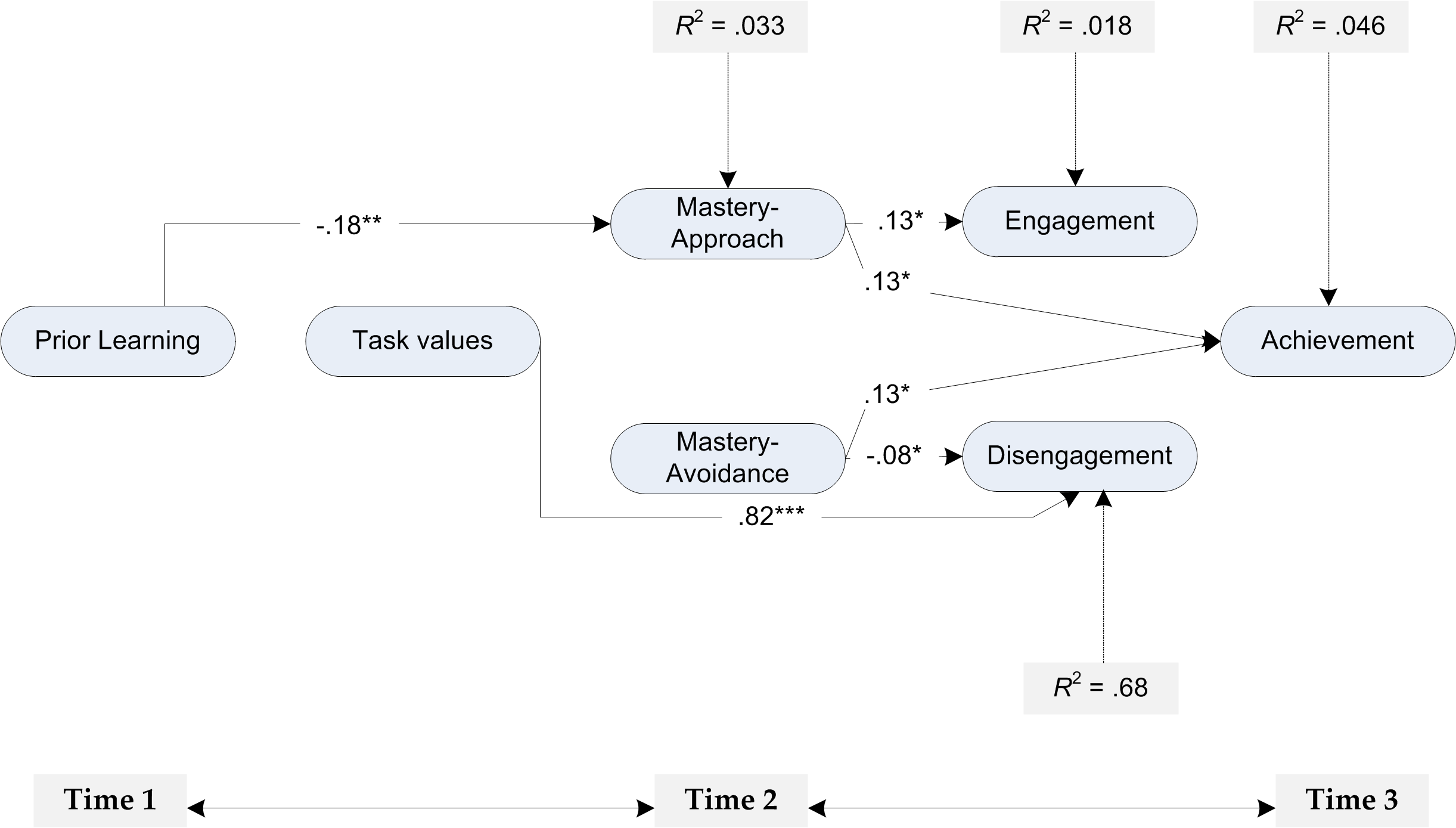
Figure 2
Final model of mastery goals, task value, and academic engagement-disengagement.
Note. Paths are statistically significant at *p < .05. **p < .01. and ***p < .001. For clarity, non-statistical significant paths have been omitted. Scores for disengagement have been reversed, so that a high score reflects more inclination towards engagement. Scores for academic disengagement (i.e., a participant less likely to disengage) have been reversed so that a positive relation for task value implies a student is more likely not to disengage. Likewise, a negative association between a mastery-avoidance goal orientation and academic disengagement implies an inverse relation.
Discussion of Results
The focus of this research study is concerned with an examination of three major theoretical orientations within one conceptual framework: task values, mastery goals, and academic engagement-disengagement. Situating within the framework of social cognition (Bandura, 1986, 1997), in particular, we explored the impact of enactive learning experience on the three mentioned constructs and academic achievement. A few notable research questions relating to the bifurcation of mastery goals and their predictive and explanatory effects (e.g., Alkharusi & Aldhafri, 2010; Elliot & McGregor, 2001; Elliot & Murayama, 2008; Van Yperen et al., 2009) on academic engagement-disengagement were considered. We used path analytical procedures to test the hypothesized structural relations depicted in Figure 1, and to discern differences pertaining to adaptive and maladaptive practices. Evidence ascertained from correlational data provides moderate support, which we discuss in this section of the article.
Table 3
Decomposition of Effects: Direct, Indirect, and Total
Predictor | Outcome | Direct | Indirect | Total | |||
---|---|---|---|---|---|---|---|
Prior learning | Task value | .013 | .000 | .013 | |||
Task value | Mastery-approach | .049 | .000 | .049 | |||
Prior learning | -.175 | ** | .001 | -.174 | ** | ||
Task value | Mastery-avoidance | .010 | .000 | .010 | |||
Prior learning | -.026 | .000 | -.026 | ||||
Mastery-approach | Academic engagement | .132 | * | .000 | .132 | * | |
Task value | -.028 | .006 | -.022 | ||||
Prior learning | .054 | -.023 | * | .031 | * | ||
Mastery-avoidance | Academic disengagement | -.075 | * | .000 | -.075 | * | |
Task value | .823 | *** | -.001 | .822 | *** | ||
Prior learning | -.064 | .012 | -.052 | ||||
Academic engagement | Achievement in mathematics | -.012 | .000 | -.012 | |||
Academic disengagement | -.072 | .000 | -.072 | ||||
Mastery-approach | .132 | * | -.002 | .130 | * | ||
Mastery-avoidance | .126 | * | .005 | .131 | * | ||
Task value | .080 | -.051 | .028 | ||||
Prior learning | -.077 | -.022 | * | -.099 | * |
*p < .05. **p < .01. ***p < .001.
The Role of Mastery Goals
An examination of the empirical literature indicates that the trichotomous model of achievement goals has received considerable research attention (e.g., Fenollar et al., 2007; Liem et al., 2008; Pekrun et al., 2009; Phan, 2010) compared to the limited emphasis of the 2 × 2 model (Elliot & McGregor, 2001; Elliot & Murayama, 2008; Sins et al., 2008; Van Yperen et al., 2009). The introduction of the bifurcation of a mastery goal in our study is significant, and yields empirical support for its structural validity. Both mastery-approach and mastery-avoidance goals influenced academic engagement and disengagement, respectively. This line of evidence, importantly, emphasizes a demarcation in consequences of both positive and maladaptive practices. Previous research has shown, for example, the positive impact of a mastery-approach goal on deep processing of information and intrinsic motivation (Elliot & Murayama, 2008; Phan, 2013c; Sins et al., 2008). In the context of this investigation, a mastery-approach goal orientation entails an inclination for students to take part in school activities, social events, etc. A mastery-avoidance goal orientation, similarly, exerted a small negative effect on disengagement. This finding seems to suggest that students who seek to avoid failing achievement of mastery tasks would incline towards disengagement (hence, the negative association with disengagement). They would likely, on this note, to withdraw from school and not take part in any learning and/or extracurricular activities.
Evidence highlighting the dichotomous effects of mastery-approach and mastery-avoidance goals (Elliot & Murayama, 2008; Sins et al., 2008; Van Yperen et al., 2009) is significant, theoretically, and for applied educational practices. Theoretically, for example, our examination emphasizes the consequences of both mastery goal types. The proximal effects of a mastery-approach goal orientation, say, would imply and suggest a number of long-term achievement-related outcomes. To what extent does a mastery-goal orientation influence students’ future time perspectives in professional growth and development? How lasting and detrimental is the effect of a mastery-avoidance goal on academic disengagement? From an educational perspective, similarly, the identification of both mastery-approach and mastery-avoidance goals as antecedents of academic engagement-disengagement suggests there are a number of considerations for educators and administrators, alike. We would want to emphasize, in particular, the saliency of respect and identification with school ethos, values, and learning outcomes. We need to consider structuring a social milieu that encourages students to adopt a mastery-approach goal orientation. Providing guidance in terms of future planning and career choices (e.g., a career day), for instance, may instil a mature mindset that enables students to consider seriously about their studies and professional trajectories. Situating learning tasks and school activities within authentic contexts may, likewise, stimulate interests, intellectual curiosities, and appreciation for mastery.
The importance of mastery goals on learning and achievement outcomes is also substantiated from our study. A mastery-approach goal orientation (e.g., striving to understand a subject matter), in this analysis, exerted a positive effect on academic achievement in mathematics. Students who strive for mastery, such as a desire to improve personal competence are more likely to learn and achieve, academically. This finding, from our point of view, is rather interesting, as some previous research studies (Elliot & Murayama, 2008; Elliot & Thrash, 2010; Phan, 2013c) have reported a null association between the two constructs. One plausible explanation, we contend, may lie in the composition of the academic achievement measure. The measure for achievement that we used, for example, entailed assessment tasks that emphasized both mastery and normative criteria. Assessment tasks that detail the saliency of competition and social comparison, in contrast, are more likely to encourage and elicit motives towards performance-based learning objectives.
We note that mastery-avoidance goals also exerted a positive effect on academic achievement. This pattern in evidence is somewhat perplexing, as it seems to suggest that students who strive to avoid an incomplete understanding of a subject matter would learn and achieve in mathematics. We reason, in this case, that other internal processes and/or extraneous factors may be at play, operating in tandem to account and predict students’ achievements. The upper secondary school years are pivotal for many students, as they prepare for post-secondary decisions and courses of action (e.g., what should I after finishing school?). No doubt, from our point of view, students would encounter difficulties, family and peer pressures to succeed, and expectations from society and others to obtain good grades. At the same time, in school and classroom settings, students would receive substantial support from teachers and administrators to succeed, academically. This combination of personal endeavours, expectations, peer pressures, and teacher support may contribute to students’ motivation for academic learning.
The impact of mastery-avoidance goals on academic achievement, as indicated, differs from previous research that showed either a null association (Elliot & Murayama, 2008; Phan, 2013c) or a deleterious effect (Van Yperen et al., 2009) between the two constructs. We concur that emphasis pertaining to the bifurcation of the mastery goal construct, according to the 2 × 2 achievement goal model, is relatively new and requires a refinement in articulations (Van Yperen et al., 2009). The mastery-avoidance goal type, in particular, as a number of researchers have alluded (Ciani & Sheldon, 2010; Elliot & Murayama, 2008; Madjar et al., 2011; Van Yperen et al., 2009), is untested and unknown in terms of its characteristics. What would of interest, in this analysis, is for researchers to consider the study of both performance-avoidance and mastery-avoidance goals. Why this avenue of inquiry and what would the focus attribute to our understanding? An examination of the literature has shown that, similarly, the effect of performance-avoidance goals is inconclusive, with some research studies reporting a null association (Fenollar et al., 2007; Liem et al., 2008; Phan, 2010; Senko & Miles, 2008; Sins et al., 2008).
The Importance of Task Values and Prior Learning Experience
It is rather interesting to note that task values only influenced academic disengagement. With the reverse in coding and subsequent statistical analyses, this inference suggests that task values influence students to engage and participate in their learning and schoolwork. Learning tasks that have relevance or perceived usefulness for future reference and/or stimulate interest and curiosity, in this sense, may motivate students to partake in a myriad of school activities, social events, and other related tasks. Difficult learning tasks that have little values and are beyond the repertoire of student knowledge, experiences, and/or interests may, in contrast, alienate and disengage students from school. This evidence contributes, theoretically, to the study student motivation, and supports the expectancy-value model of learning (Eccles, 2005; Eccles et al., 1983; Wigfield & Eccles, 2000). From an educational perspective, similarly, an emphasis on task values could be made to discourage academic disengagement. We could specifically emphasize and encourage the various types of subjective task values in classroom learning (e.g., perceived usefulness). Focusing and explaining the potential relevance of a subject matter (e.g., mathematics) towards future time perspectives (e.g., a career choice) may, for example, stimulate and instil serious consideration from students. Students may realize the importance and perceived usefulness of academic learning, thereby making more effort to participate in school activities, social events, etc.
Enactive learning experiences, consistent with social cognitive theory (Bandura, 1986, 1997), influenced a mastery-approach goal orientation. Interestingly, however, students’ previous performance accomplishments, subject to both mastery criteria and competitive evaluation and social comparison, were found to exert a negative effect on a mastery-approach goal orientation. Academic success in mathematics, in this analysis, is more likely to result in students opting not to strive for in-depth understanding a mastery of this subject matter. We query whether this inversed association could have been the result of misalignment between students’ prior learning and their subsequent consideration of academic aims, motives, etc. The reporting of individuals’ self-ratings of Likert-scale inventories and surveys in psychological and social sciences research has been observed to restrict to single method variance and other limitations (Fulmer & Frijters, 2009; Podsakoff, MacKenzie, & Podsakoff, 2012). The inaccurate judgements of students’ perceptions of their goals and subsequent reporting may inflate and cause erroneous responses. In the area of personal self-efficacy (Bandura, 1977, 1997), specifically, researchers have coined the term ‘calibration’ (Pajares, 1996; Pajares & Kranzler, 1995; Pajares & Miller, 1994), which depicts the state of overconfidence or underconfidence in one’s self-efficacy beliefs for academic learning. This inaccuracy, in turn, misaligns with one’s own actual capability to solve a set of problems, etc.
In a similar vein, apart from prior learning experience and task values, there may be other extraneous factors and/or internal processes that could account for the overall variance of mastery goal orientations. Elliot and Murayama’s (2008) study, for example, showed that a need for achievement (e.g., "I enjoy difficult work": Jackson, 1974) influenced both mastery-approach (β = .34, p < .01) and mastery-avoidance (β = .21, p < .01) goal orientations. The question then, taking into consideration this evidence, is whether enactive learning experience could co-exist with other motivational variables to influence mastery goal orientations. The social milieu at large, as indicated by a number of researchers (e.g., Ames, 1992; Anderman & Midgley, 1997; Urdan, 2004; Urdan, Kneisel, & Mason, 1999), also features predominantly in students’ learning. Structuring a classroom environment that emphasizes the saliency of a mastery goal orientation (e.g., mastery-approach goal type) may encourage a preference for this type of goal over the course of time.
The Central Role of Mastery Goals
The decomposition of direct and indirect effects in this study highlights the central role of a mastery-approach goal orientation. This preliminary evidence is rather unique and aligns closely to the work of Elliot and Murayama (2008), which illustrated the central role of a mastery-approach goal between a need for achievement and intrinsic motivation. Elliot and Thrash’s (2010) recent study, in contrast, yielded a null central role for a mastery-approach goal between approach temperament (e.g., "Thinking about the things I want really energizes me": Elliot & Thrash, 2010) and exam performance. For a mastery-avoidance goal, in contrast, we note that our findings, indicating a non-statistical significance, are similar to those obtained in previous studies (Elliot & Murayama, 2008; Elliot & Thrash, 2010). Elliot and Murayama (2008) found, for instance, that there was a lack of a central role for a mastery-avoidance goal between fear of failure (e.g., "When I am failing, I worry about what others think about me": Conroy, 2001) and intrinsic motivation or exam performance. A performance-avoidance goal, however, served as potential mediator between fear of failure and intrinsic motivation and final exam performance.
In relation to the trichotomous model of achievement goals, there is also consistent evidence to emphasize the central role of mastery goals (Durik et al., 2009; Greene et al., 2004; Harackiewicz et al., 2008; Liem et al., 2008; Phan, 2009; e.g., Simons et al., 2004). This evidence, in conjunction with recent findings on the 2 × 2 model (Elliot & Murayama, 2008; Elliot & Thrash, 2010), indicates the importance in relations between antecedents and consequences of achievement goals. This depiction acknowledges the fact that achievement goals, such as mastery-approach and mastery-avoidance do not exist in isolation.
Caveats and Consideration for Future Research
The current study, in general, has yielded some notable evidence worth considering for continuing research development and educational practices. The final solution reported for discussion, as depicted in Figure 2, illustrates the intricate interrelations between the theoretical constructs that originate from students’ enactive learning experiences. We note, however, that despite the empirical validation of some hypothesized structural relations, there are also a few major limitations that warrant further examination. These limitations, from our point of view, establish grounding for continuing research development into this area of cognition and motivation of student learning.
Firstly, the methodological design adopted for this investigation has limited us from making sound statistical inference about the directions of causality between the variables involved (Marsh & Yeung, 1997, 1998). The task values – mastery goal relation, for example, is subject to different theorizations and interpretations. Hulleman et al. (2008) found, for example, that a mastery-approach goal orientation was predictive of both intrinsic and utility values, which in turn influenced subsequent interest and final grade. This pattern differs from other findings (Greene et al., 2004; Harackiewicz et al., 2008; e.g., Liem et al., 2008) that showed the impact of task values (e.g., task interest) on various types of achievement goals. With this dilemma in mind, it would be of considerable interest for educators and researchers to consider the use of multi-wave panel data (Marsh & Yeung, 1997; Martin, Colmar, Davey, & Marsh, 2010; Rogosa, 1979), analysed within the framework of SEM to provide empirical and theoretical insights into this causal predominance relationship.
Secondly, the longitudinal design used for this study was rather short-term, and does not provide detailed information about the possible changes that could take place in the upper secondary school years. A duration of 6 to 7 months across three time points, in our view, is too brief to detect and attest to major changes or causal, long lasting effects of variables. Researchers could therefore consider longer time periods, in multi stages, with data analyzed using latent growth modelling (LGM) procedures (Bollen & Curran, 2006; Duncan, Duncan, Strycker, Li, & Alpert, 1999; McArdle & Nesselroade, 2003). This complex methodological approach, increasingly being used in education and social sciences research studies (Caprara et al., 2008; Geary, 2011; Gutman, Sameroff, & Cole, 2003; Phan, 2013a), is more stringent and sophisticated, enabling researchers to explore and identify internal cognitive processes and/or extraneous factors that could account for the initial state and rate of change of psychological variables.
Thirdly, we limited our investigation to academic engagement-disengagement and achievement in mathematics. We contend, however, that adaptive outcomes espouse more than just academic achievement. Researchers could, in this analysis, consider other non-academic outcomes, which may also contribute to personal makeup and the social fabrics of a nation. It would be of theoretical interest, for example, for us to explore the impact of academic engagement, say, on personal well-being and social relationship. For example, does disengagement influence an individual’s relationship with family members, etc.? In a similar vein, it would be of significance to explore the impact of the mastery-approach goal – engagement relation on other future achievement-related outcomes, such as career choices, civics citizenship, and social initiatives. The impact of academic engagement on students’ proactive relations with a local community, for instance, may provide fruitful information for educators to consider. The potential detrimental effects of academic disengagement (e.g., substance abuse) may, similarly, highlight the failings of school policies, pedagogical practices, etc.
Fourthly, the sample used in the present investigation was modest in size. Consequently, this sample size limited us from exploring other theoretical constructs and interrelationships. The importance of the 2 × 2 model (Elliot, 1999; Elliot & McGregor, 2001; Harackiewicz, Barron, Pintrich, et al., 2002; Pintrich, 2000), for example, has formed the basis for educators and researchers to consider different achievement goal orientations in educational contexts. Future research could expand our theoretical-conceptual model and explore, comparatively, the effects of the four achievement goal types (e.g., mastery-approach versus performance-approach). One emphasis for consideration, in particular, involves the validation of structural relations between academic achievement and performance-avoidance and mastery-avoidance goals.
Finally, our focus of examination involved mathematics, an academic subject that features prominently in academia for a number of achievement and decision-related purposes (e.g., prerequisite to entry into a degree program). Other academic subjects and disciplines may not necessarily espouse the same epistemological beliefs and/or thinking (Becher, 1987, 1994). We ponder whether the theoretical-conceptual model outlined in the present study would apply and cross-generalize to other academic subjects. The importance of academic engagement in, say, English or History would be of an interest for educators to advance. This line of inquiry, from our point of view, may provide an in-depth understanding into facets that constitute a unit of study, and whether these would explain or enhance students’ engagement. In a similar vein, apart from subject domains of functioning, it is of relevance for future research to consider the impact of extraneous influences on students’ motivational beliefs and engagement. Historical upbringing and sociocultural attributes and customs may, for example, influence students’ motives for learning and achievements. Education may, in this instance, serve as a basis for social and economical mobility, motivating students to engage proactively in their academic studies.